The Three C's of Big-Data Applications | Intel Science & Technology …
By David Maier, Portland State University
If you are reading this blog, then you’ve already heard of the Three V’s of Big Data (Volume, Velocity and Variety). While the V’s are a way to characterize Big Data, I’ve been thinking about what enables new applications of Big Data. Why is a certain application appearing now, rather than earlier or later? I’ve come up with the Three C’s of Big-Data applications: Cost, Coverage and Currency. I’ll explain each in turn, and try to illustrate.
Cost: Projected revenue or savings must cover the predicted expenditures for collection, storage and analysis of data for the application. Thus, a new Big-Data application might be undertaken because the cost of sensors, storage or computation has dropped sufficiently to make it economical.
An example here is the problem of assortment optimization: determining the optimal selection and inventory of items to stock at different outlets of a retail entity. Perhaps the 7-Eleven at a subway stop should carry umbrellas, whereas they wouldn’t be good sellers at a suburban location where everyone arrives by car. I’ve seen estimates that good assortment optimization can lead to a 2% increase in sales. Given that added profit might only be a small fraction of sales, a company can’t spend huge amounts to collect the needed sales and demographic data, store it until there’s an adequate amount to analyze, run the optimization algorithms, and still expect to get a net benefit. However, with some forms of cloud storage priced at $10/TB/month, and cluster rental running as low as 5¢/hour/node, the break-even point for assortment optimization is dropping lower and lower.
Coverage: The data set is sufficiently complete in time, space and kind for reasonable application performance. An application is unlikely to be successful if there are significant gaps in the input it needs to produce decent results.
Companies have started offering various forms of a walkability index for residential properties. Such an index rates locations as to how easily one can meet the needs of daily life on foot. I believe such applications are emerging now because of sufficient coverage of the input parameters for the index. For most cities, nearly complete information is available on road networks, tax lots and transit routes. And there is more or less complete information available on destinations: stores, dining, schools. In fact, the critiques I’ve seen of different walkability indices are often from a coverage standpoint: they don’t have sufficient information on the presence of sidewalks and crossings, or particulars of destinations such as store size.
Currency: The application can receive the information it requires in time to react. How soon enough is soon enough depends of course on the nature of the application domain. For algorithmic trading, microseconds can make a difference, and electronic exchanges make money renting out space on site for traders to co-located servers.
For other applications, getting data within 5 or 10 minutes might be soon enough. The CitySense product (from Sense Networks) leverages positional data collected by mobile devices (both historical and current) and knowledge of venues to find places that have more people than usual at the current time. While CitySense uses this information to highlight activity hot spots, I can imagine other uses, such as dispatching taxis to the area, siting pop-up retail, or even emergency evacuation.
Are there other C’s that enable Big-Data applications? Correctness? Connection? I don’t have a good example yet of where data becoming more accurate, or a new way to link data, has been the key enabler of an application, but I’d love to hear of one. What do you think?
Originally from:
The Three C's of Big-Data Applications | Intel Science & Technology …
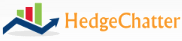